Unlocking AI Potential: The Importance of Labeling Images for Object Detection
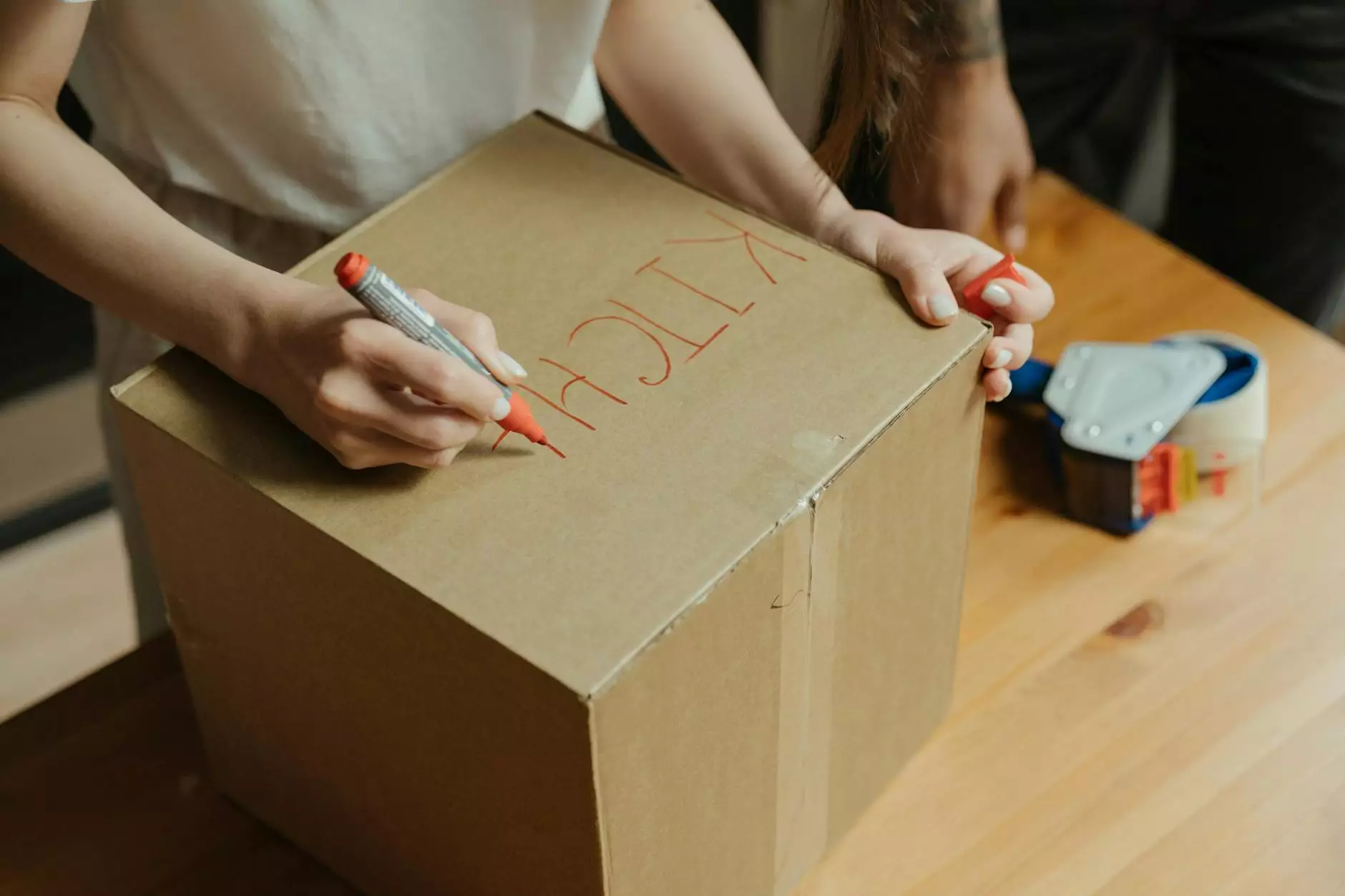
In the rapidly evolving world of artificial intelligence and machine learning, one of the crucial tasks at the forefront is to accurately label images for object detection. This task forms the backbone of numerous AI applications, enabling machines to recognize and interpret visual data effectively. Understanding this pivotal role can significantly enhance your ability to develop robust AI solutions.
The Importance of Object Detection in Today’s Technology Landscape
Object detection is a vital area in computer vision that involves identifying and localizing objects within images or videos. It has numerous applications, including:
- Autonomous Vehicles: Utilizing object detection allows self-driving cars to interpret their surroundings effectively.
- Surveillance Systems: Enhancing security by detecting and tracking individuals in real-time.
- Retail Analytics: Analyzing customer behavior and interactions within stores.
- Healthcare Imaging: Supporting diagnostics by identifying conditions in medical scans.
Each of these applications relies heavily on high-quality labeled data. Flawless labeling is not just a best practice; it is a necessity for achieving exemplary performance in AI-driven systems.
Understanding Data Annotation: The Process Behind Effective Object Detection
The process of object detection begins with data annotation, which involves creating datasets that enable machine learning models to learn from examples. Labeling images for object detection encompasses various steps:
- Image Selection: Carefully choosing representative images that cover a wide range of scenarios.
- Defining Labels: Establishing the categories to be identified in the images, such as bicycles, cars, or pedestrians.
- Annotation Techniques: Applying various techniques like bounding box labeling, polygon annotation, or instance segmentation.
- Quality Control: Reviewing labeled data to ensure accuracy and consistency.
In each step, it is essential to maintain a high level of precision, as errors in the labeled data can lead to significant inaccuracies in AI models.
Types of Image Annotation Techniques for Object Detection
Several annotation techniques can be employed to label images effectively. Each method has its unique use cases and advantages:
1. Bounding Box Annotation
This is the most common method used for object detection. It involves drawing rectangular boxes around the objects of interest within images. This technique is fast and straightforward, making it suitable for datasets with many objects.
2. Polygon Annotation
Polygon annotation allows annotators to create more precise labels by outlining the exact shape of an object. This is particularly useful for irregularly shaped objects and provides better training data for complex scenes.
3. Semantic Segmentation
In semantic segmentation, each pixel in an image is classified as belonging to a particular object class. This technique offers a fine-grained understanding of an image and is beneficial when dealing with multiple overlapping objects.
4. Instance Segmentation
Combining the benefits of bounding box annotation and semantic segmentation, instance segmentation identifies each object instance distinctly. This allows for more nuanced detection within heavily populated scenes.
The Role of KeyLabs.ai in Data Annotation
KeyLabs.ai provides a state-of-the-art platform designed to facilitate effective image labeling for object detection. Our tools streamline the process, ensuring that businesses can harness accurate annotation without the overhead of managing multiple tasks separately.
Features of KeyLabs.ai’s Data Annotation Tools
- User-Friendly Interface: Our platform is designed for ease of use, allowing teams to quickly adapt and start labeling images.
- Collaboration Tools: Enhanced team collaboration features enable multiple users to work on projects seamlessly, ensuring efficiency.
- Quality Assurance Protocols: Built-in quality checks uphold the highest standards in data accuracy. Every piece of labeled data undergoes rigorous review processes.
- Scalability: Whether you need to annotate thousands of images or millions, our platform scales according to your needs.
Why Choosing Quality Annotation Matters
The quality of your labeled data directly affects the performance of your AI models. Poorly labeled images can lead to failing to generalize to real-world scenarios, resulting in models that produce inaccurate predictions or do not perform reliably in critical applications.
By partnering with a platform like KeyLabs.ai, you can ensure that your data annotation is handled professionally, enhancing the reliability of your machine learning models. With accurate and high-quality labeled images, businesses can realize:
1. Improved Model Accuracy
High-quality labels lead to models that learn effectively, improving their predictive accuracy and performance in real-world applications.
2. Faster Deployment of AI Solutions
Efficient annotation processes can dramatically reduce the time taken from data collection to deployment, allowing businesses to capitalize on AI-driven insights sooner.
3. Cost-Effective Solutions
By utilizing an efficient data annotation tool, organizations can reduce costs associated with manual labeling while maintaining quality. This optimizes resource allocation and improves ROI on AI projects.
Conclusion: Embracing the Future with Quality Image Labeling
Labeling images for object detection is a foundational element in the realm of AI and machine learning. As technology continues to evolve, the demand for quality data becomes even more pronounced. By investing in a robust data annotation platform like KeyLabs.ai, organizations can ensure they remain competitive in the fast-paced digital landscape.
Embrace the power of advanced labeling techniques, and unlock the true potential of your AI initiatives. With our tools, you can ensure your models are built on a foundation of accurate, high-quality labeled data, driving your success in object detection and beyond.